Edellisessä postauksessa tein ensimmäisiä hakuja Europeanan SPARQL-palveluun. Kiitos kuuluu Bob DuCharmelle, jonka selkeillä ohjeilla pääsi alkuun. Sittemmin olen tutkinut Europeanaa lisää. Vallan mainiota, että tällainen yhteiseurooppalainen ponnistus on tehty. Rahaa on käytetty hullumminkin. Antoine Isaac ja Bernhard Haslhofer kirjoittavat artikkelissaan Europeana Linked Open Data – data.europeana.eu (PDF):
Europeana is a single access point to millions of books, paintings, films, museum objects and archival records that have been digitized throughout Europe. The data.europeana.eu Linked Open Data pilot dataset contains open metadata on approximately 2.4 million texts, images, videos and sounds gathered by Europeana. All metadata are released under Creative Commons CC0 and therefore dedicated to the public domain. The metadata follow the Europeana Data Model and clients can access data either by dereferencing URIs, downloading data dumps, orexecuting SPARQL queries against the dataset.
Pilotti tarjoaa runsaasti materiaalia mm. SPARQL-kyselyjen treenaamiseen, ei vähiten siksi että metadatamalli on aika mutkikas. Pakko sanoa, että ilman Bobin virtuaalista kannustusta olisin tuskin tohtinut edes yrittää. Kehittäjät tunnustavat tilanteen konferenssiesitelmässä data.europeana.eu, The Europeana Linked Open Data Pilot (Dublin Core and Metadata Applications 2011, The Hague):
Beyond adding extra complexity to the RDF graphs published, the proxy pattern, which was introduced because of the lack of support for named graphs in RDF, is indeed quite a counter-intuitive necessary evil for linked data practitioners — including the authors of this paper […] We were tempted to make the work of linked data consumers easier, at least by copying the statements attached to the provider and Europeana proxies onto the “main” resource for the provided item, so as to allow direct access to these statements—i.e., not mediated through proxies. We decided against it, trying to avoid such data duplication. Feedback from data consumers may yet cause us to re-consider this decision. On the longer term, also, we hope that W3C will soon standardize “named graphs” for RDF. This mechanism would allow EDM to meet the requirements for tracking item data provenance without using proxies. (s. 100)
Named graphs -käsitteestä tarkemmin ks. Wikipedia. Kotimainen esimerkki nimettyjen graafien toteutuksesta on Aalto-yliopiston Linked Open Aalto.
Finlandia-katsaus 263
Otetaan esimerkkivideo, Kansallisen Audiovisuaalisen Arkiston (KAVA) Finlandia-katsaus 263 vuodelta 1955. Europeanan RDF-tietovarastossa siitä on tallennettu metatietoa kahteen ore:Proxy -solmuun. Toisessa on datan toimittajan (provider) eli KAVAn antamaa tietoa, toisessa Europeanan. Europeanan solmusta löytyvät mm. kaikki sen tekemät lisäykset (enrichments) alkuperäiseen metatietoon, kuten linkitykset KAVAn kertomasta dcterms:created -vuosiluvusta Semium-sanastolla ilmaistuun aikaan ja dc:spatial -paikannimestä GeoNames-tietokantaan. Datan alkuperätiedot (provenance) ovat ore:ResourceMap -solmussa.
Missä itse video sitten on? Sen selvittämiseksi pitää käydä koontisolmussa. Niitäkin on kaksi: datan toimittajan ore:Aggregation ja Europeanan edm:EuropeanaAggregation. Esimerkkivideon ore:Aggregation -tiedoista selviää videon kotisivu edm:isShownAt ja MP4-tiedosto edm:isShownBy. edm:EuropeanaAggregation kertoo videon sivun Europeanan web-portaalissa edm:landingPage.
soRvi
SPARQL-kyselykielen lisäksi olen jo jonkin aikaa opiskellut R-ohjelmointikieltä. Yksi viime vuosien R-tapauksia Suomessa on ollut avoimen datan työkalupakki soRvi. Päätin kokeilla, miten työskentely sillä sujuu. Tavoite pitää olla: Suomen kartta, jossa väri ilmaisee paljonko Europeanassa on kuntiin liittyviä videoita.
Sorvilla saa kätevästi Suomen kuntien nimet ja kuntarajat. Data tulee Maanmittauslaitokselta (MML). Entä Europeana? Miten nimet on siellä esitetty ja missä? Kahlasin portaalin avulla läpi joukon videoita, ja katsoin metatietoelementtejä sivun lähdekoodissa. Esimerkkivideossa Finlandia-katsaus 263 on useampikin pätkä Helsingistä. Helsinki-sana löytyy perusmuodossa kentistä dc:subject ja dc:description, englanninkielisestä käännöksestä. Muutamissa videoissa näkyi dc:spatial ja sen myötä Europeanan lisäämä GeoNameID. Lisäksi nimi voi esiintyä paitsi varsinaisessa otsikossa dc:title myös vaihtoehtoisessa otsikossa dcterms:alternative (en tiedä miksi).
Suomen kuntien nimissä on runsaasti äännevaihtelua ja taipumista. Syntymäkuntani Laitila ei taivu, mutta nykyinen kotikaupunkini Helsinki taipuu. Kun katsoo kuntaluetteloa, silmissä vilisee lahtia, järviä, lampia, koskia ja jokia. Välissä on kuivaakin maata kuten rantoja, saaria, mäkiä ja niemiä. Taipuvia kaikki.
Rajoitin haut nimen perusmuotoon sillä lisäyksellä, että jos säännöllinen lauseke löytää taipumattomien nimien päätteellisiä muotoja (Oulu, Oulun, Oulussa jne.), hyvä niin. Tällä periaatteella on ilmiselvä kääntöpuolensa. Lyhyet kuten Ii ja Salo tulevat tuottamaan vääriä hakutuloksia sekä Suomesta että muista maista. Ii saa omiensa lisäksi myös Iisalmen ja Iitin videopinnat, mikä on ehkä oikein ja kohtuullista kunnalle, jolla on vain kaksi kirjainta. Salo-kirjainyhdistelmää esiintyy paitsi suomessa myös ainakin tanskassa, ranskassa, katalaanissa ja italiassa.
Tein sen minkä voin ja rajasin haun vain niihin videoihin, joiden dc:language on fi. Tämä päätös tiputtaa kuitenkin tuloksesta pois ulkomaista alkuperää olevat videot jotka todella liittyvät Suomeen ja ne, joissa tätä Dublin Core -elementtiä ei ole annettu. Toisaalta suomenruotsalaisten kuntien hakutulos siistiytyy, sillä oletettavasti haaviin ei näin jää Ruotsin samannimisiä kuntia.
Kartalla
Kuntakartan plottaus absoluuttisilla luvuilla kävi helposti soRvi-blogin esimerkkien avulla. Jouduin tosin jättämään Helsingin kokonaan pois, jotta muut kunnat pääsivät esille. Data vaatisi oikeastaan logaritmisen asteikon; Helsinki poikkeaa niin paljon muista.
Ensimmäisessä kartassa kunnat ilman Helsinkiä, toisessa ne kunnat joihin liittyviä videoita löytyi enintään 20.
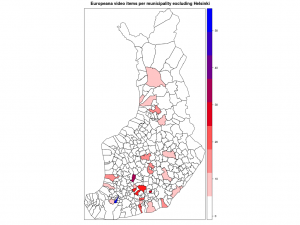
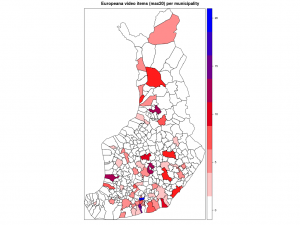
Alla matkin suoraan sitä, miten Datavaalit havainnollisti ahkerimpia sosiaalisen median käyttäjiä.
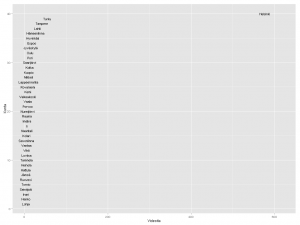
Kärkikolmikko ei yllätä: Helsinki, Turku ja Tampere. Ystävämme Ii yltää 25 ensimmäisen joukkoon. Pääkaupunkiseudun nykyisistä isoista kaupungeista Vantaalla näyttäisi olevan videoita vain muutama. Vantaasta tuli kuitenkin kunnan nimi vasta 1970-luvulla, ja uusimmat Europeanan videot ovat nähtävästi 1960-luvulta. Vantaa viittaakin näissä Vantaanjokeen. Hyvinkään lukua selittää mm. Kone Oyj ja Herlinin suku. Tunisian presidentti Bourgiba vieraili 1960-luvun alussa Herlineillä.
Suhteellista
Seuraavaksi suhteutin videoiden määrän kunnan asukaslukuun. Sorvi tarjoaa valmiin funktion, joka hakee asukasluvut suoraan Tilastokeskuksesta. Vuoden 2013 alusta lukien kuntien määrä väheni vajaalla 20:lla kuntaliitosten myötä. Kunnat ja kuntarajat kuvaavat tässä kuitenkin mennyttä aikaa, vuotta 2012. Lisäsin entisille kunnille asukasmäärän käsin, mutta niiden kuntien lukuun en koskenut, joihin nämä kunnat yhdistettiin.
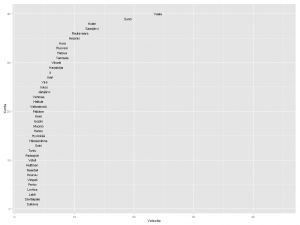
Nyt erottuvat suuruusjärjestyksessä Vaala, Sund, Kolari, Rautavaara ja Helsinki. Moni on kuitenkin väärä positiivinen. Kai Sundström -nimistä henkilöä videoitiin kahteen otteeseen 1940-luvulla. Näin ollen algoritmini antoi kaksi videopistettä pienelle ahvenanmaalaiselle Sundin kunnalle. Kolarin asema johtuu vain ja ainoastaan otsikoista Kolari Helsingissä. Tapio Rautavaara taas oli 50-luvulla julkisuuden henkilö monella alalla, itse Rautavaaran kunnasta ei videoita löydy. Mutta entä Vaala? Tämä reilun 3000 asukkaan kunta Kainuussa on vanhaa asutusaluetta, mutta sen lisäksi myös sukunimikaima elokuvaohjaaja Valentin Vaalalle.
Sivumennen sanoen opin Wikipediasta, että sana vaala liittyy sekin veteen. Englanninkielinen Wikipedia-artikkeli mainitsee, että se on the phase in a river just before rapids.
Helsinki on siis väkilukuunkin suhteutettuna videoykkönen. Seuraavana tulevat oikeat videokunnat Karjalohja ja Vihanti. Suomi-Filmi videouutisoi näistä kunnista politiikan ja talouden näkökulmasta. Pääministeri Edwin Linkomiehen kesäpaikka oli Karjalohjalla, ja Vihantiin rakennettiin 1950-luvun alussa valtion toimesta rautatie. Outokumpu Oyj perusti Vihantiin sinkkirikastekaivoksen. Kaivos toimi vuosina 1954-1992, tietää Wikipedia ja jatkaa:
Kaivoksen tuotantorakennukset purettiin pari vuotta myöhemmin ja kaivostorni räjäytettiin. Myös kaivokselta Vihannin asemalle vienyt junarata on purettu Vihannin päässä olevaa 1,5 kilometrin pituista vetoraidepätkää lukuunottamatta. Kaivoksen toimistorakennukset säilytettiin. Osa kaivosalueesta on aidattu sortumavaaran vuoksi.
Vihannin kuntaa ei enää ole. Se liitettiin vuoden 2013 alusta Raaheen.
Paikan haku
GeoNames-tietokanta vaikuttaa lupaavalta. Ajattelin jo nyt hyödyntää geonames R-kirjastoa kuntien GeoNameID:n selvittämiseen, mutta en päässyt alkua pidemmälle. Palvelu kyllä vastaa ja palauttaa dataa. Liikaakin, aloittelijalle. Kysely on ilmeisesti rakennettava hyvinkin yksityiskohtaisesti kohdistumaan vain tietyntyyppisiin taajamiin.
Yritin myös ujuttaa SPARQL-kyselyyn soRvin tarjoamia MML:n kuntakoordinaatteja. Europeanan SPARQL-editorissa on valmis esimerkki Time enrichment statements produced by Europeana for provided objects. Se antaa kuitenkin ymmärtää, että metatieto-rikastukset mm. ajalle ja paikalle olisivat toistaiseksi haettavissa vain yleisellä merkkijonohaulla, joten luovutin.
Tuore Europeana Business Plan 2013 kertoo tammikuun tilanteen paikkatiedoista. Ne löytyvät 27.5 prosentissa kaikesta aineistosta.
Paljonko Europeanassa sitten on Suomen GeoNameID:llä <http://sws.geonames.org/660013/> varustettuja RDF-kolmikkoja resurssityypeittäin (image, sound, text, video) ja lähteittäin? Kopioi tästä kysely, liimaa SPARQL-editoriin ja lähetä.
Dataa ja videonauhaa
Linkitetty avoin data on Europeana-pilotti. Datanarkkarille se tarjoaa mahdollisuuden ynnäillä vaikka tilastoja, mutta ne ovat vain sivutuote. Datan päätarkoitus on kypsyttää ideoita verkkopalveluiksi. Liikkuvalla kuvalla ja äänellä on kysyntää. Niitä aiotaankin saada lisää, linjaa Business Plan:
Actively pursue both large and small institutions to contribute AV material through national aggregators or audiovisual projects. AV material currently makes up less than 3% of the database, while research shows that this material gets most attention from end-users. (s. 9)
R-koodi.
EDIT 16.3.: Missä mahtoivat silmäni olla, kun katsoin asukaslukuun suhteutettua tilastoa? En osaa selittää. Oli miten oli, Helsinki ei suinkaan ole videoykkönen vaan Saarijärvi! Lisäksi Vihannin ja Karjalohjan ohittavat Aura, Ruovesi, Halsua ja Tammela. Aura on tosin siinä ja siinä, koska toinen kahdesta videosta liittyy Teuvo Auraan.