Kulosaari (Brändö in Swedish), an 1,8 square km island in Helsinki, detached itself from the Helsinki parish in early 1920’s, and became an independent municipality. The history of Kulosaari is an interesting chapter of Finnish National Romantic architecture and semi-urban development. It all began in 1907 when the company AB Brändö Villastad (Wikipage in Finnish) was established – but that’s another story. In 1949, the island was annexed again by Helsinki. Today, Kulosaari is cut in half by one of the busiest highways in Finland. The idealistic, tranquil village community is long gone. Since late 1997, Kulosaari has been my home suburb.
One of the open datasets provided by Helsinki Region Infoshare, is a scanned map of Kulosaari from 1917. Or rather, a scheme which became reality only in a limited extent. As long as I’ve known a little about what georeferencing is all about – thanks to the excellent Coursera MOOC Maps and the Geospatial Revolution by Dr. Anthony C. Robinson – I’ve had in mind to work with that map some day. That day dawned when I happened to read the blog posting Using custom tiles in an RStudio Leaflet map by Kyle Walker.
Unlike Kyle, I haven’t got any historical data to render upon the 1917 map but instead, there are a number of present day datasets available, courtesy of the City of Helsinki, e.g. roadmap and 3D models of buildings. How does the highway look like on top of the map? What about buildings and their whereabouts today? Note that I don’t aim particularly high here, or to more than two dimensions anyway; my intention is just to get an idea of how the face of the island has changed.
Georeferencing with QGIS is fun. I’m sure there are many good introductions out there in various languages. For Finnish speakers, I can recommend this one (PDF) by Latuviitta, a GIS treasure chamber.
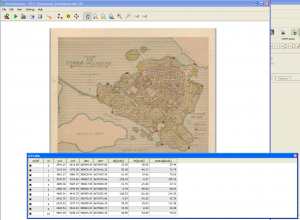
The devil is in the detail, and I know I could’ve done more with the control points, but that’s a start. When QGIS was done with number-crunching, the result looked like this when I adjusted transparence for an easier quality check.
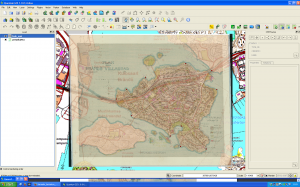
Not bad. Maybe hanging a tad high, but will do.
Next, I basically just followed Kyle’s footsteps and made tiles with the OSGeo4W
shell. I even used the same five zoom layers than he. Then I uploaded the whole directory structure with PNG files (~300 MB) to my web domain where this blog resides, too.
Roadmap data is available both in ESRI Shapefile and Google KML. I downloaded the zipped Shapefile, unzipped it, and imported as new vector layer to QGIS. After some googling I found help on how to select an area – Kulosaari main island in my case – by rectangle, how to merge selected features, and how to save the selection as a new Shapefile.
Then, to RStudio and some R code.
In Kulosaari, there are 23 different kind of roads. Even steps (porras) and boat docks (venelaituri) are categorized as part of the city roadmap.
> unique(streets$Vaylatyypp)
[1] "Asuntokatu" "Paikallinen kokoojakatu"
[3] "Huoltoajo sallittu" "Moottoriväyläramppi"
[5] "Alueellinen kokoojakatu" "Silta tai ylikulku (katuverkolla)"
[7] "Moottoriväylä" "Pääkatu"
[9] "Silta tai ylikulku (jalkakäytävä, pyörä "Alikulku (jalkakäytävä, pyörätie)"
[11] "Jalkakäytävä" "Porras"
[13] "Yhdistetty jalkakäytävä ja pyörätie" "Puistotie (hiekka)"
[15] "Ulkoilureitti" "Puistokäytävä (hiekka)"
[17] "Puistokäytävä (päällystetty)" "Venelaituri"
[19] "Polku" "Suojatie"
[21] "Väylälinkki" "Pyöräkaista"
[23] "Pyörätie"
From these, I extracted motorways, bridges, paths, steps, parkways, streets allowed for service drive, and underpasses.
Working with the 3D data wasn’t quite as easy (no surprise). By far the biggest challenge turned to be computing resources.
I decided to work with KMZ (zipped KML) files. The documentation explained that the data is divided into 1 x 1 km grids, and that the numbering of the grids follows the one used by Helsingin karttapalvelu (map service). The screenshot below shows one of the four grids I was mainly interested in: 675499 (NW), 674499 (SW), 675500 (NE) and 674500 (SE). These would leave out outer tips of the island in the East, and bring in a chunk of the Kivinokka recreation area in the North.
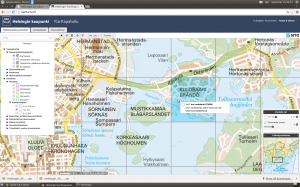
First I had in mind to continue using Shapefiles: imported one KML file to QGIS, saved as Shapefile, and added it as a polygon to the leaflet map. It worked, but I noticed that RStudio started to slow down immediately, and that the map in the Viewer became seemingly harder to manipulate. How about GeoJSON instead? Well, the file size do was reduced but still too much data. Still, I succeeded in getting all on the map, of which this screenshot acts as the evidence:
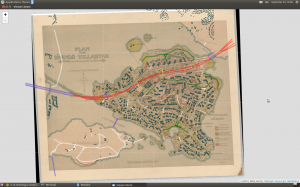
However, where I failed was to get the map transformed to a web page from the RStudio GUI. The problem: default Pandoc memory options.
Stack space overflow: current size 16777216 bytes.
Use `+RTS -Ksize -RTS' to increase it.
Error: pandoc document conversion failed with error 2
People seem to get over this situation by adding an appropiate command to the YAML metadata block of the RMarkdown file, but I’m not dealing with RMarkdown here. Couldn’t get the option work from the .Rprofile
file either.
Anyway, here is the map without the buildings, so far: there is the motorway/highway (red), few bridges (blue), sandy parkways (green) here and there, a couple of underpasses (yellow), streets for service drive only (white) – and one path (brown) on the Southern coast of the neighbour island Mustikkamaa, as unbuilt as in 1917.
Note that interactivity in the map is limited to zooming and panning. No popups, for example.
I’ve heard many stories of the time when the highway was built. One detail mentioned by a neighbour is also visible on the map: it reduced the size of the big Storaängen outdoor sports area on the Southern side of the highway. The sports area is accessible from the Hertonäs Boulevarden – now Kulosaaren puistotie – by an underpass.
EDIT 26.3.2015: Thanks to the helpful comment by Yihui Xie, I realized that there is in fact several options to do a standalone HTML file from the RStudio GUI. With File > Compile Notebook...
the result was combiled without problems, and now all buildings are rendered in the leaflet too. The file is a whopping 7 MB and therefore slow in its turns, but at least all data are now there. As a bonus, the R code is included as well! RStudios capabilities don’t stop to amaze me.